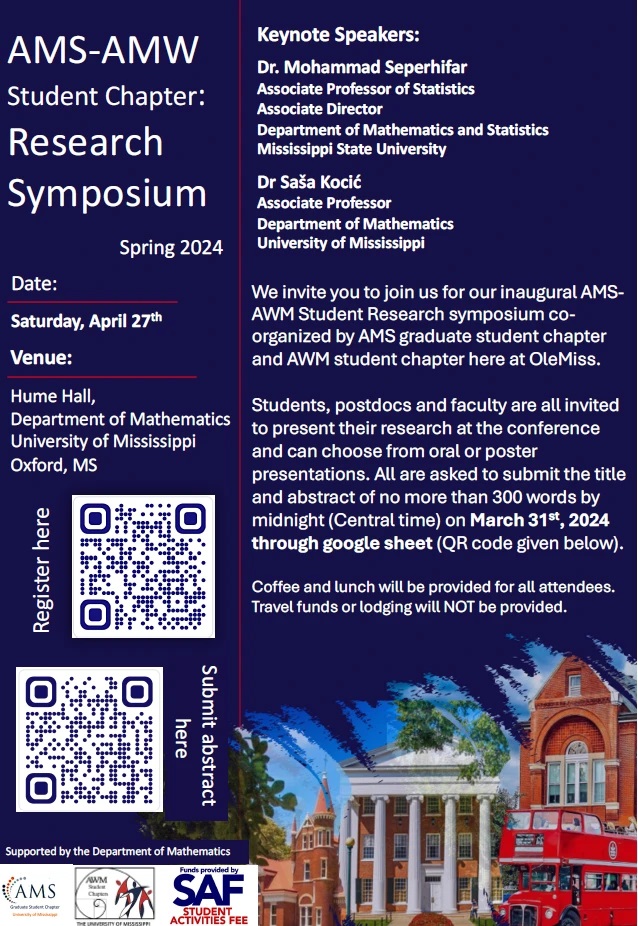
Weighted Average Iterated filtering using p-generalized Gaussian smoothing
Location
Room 321, Hume Hall
Start Date
27-4-2024 10:00 AM
End Date
27-4-2024 11:00 AM
Description
In recent years, simulation-based inferences have garnered significant attention due to the inherent challenges in directly computing likelihood functions for many real-world problems. Iterated f iltering Ionides et al. (2006) has emerged as a method to maximize likelihood functions by perturbing models and approximating the gradient of log-likelihood through sequential Monte Carlo f iltering. Using Stein’s identity, Doucet et al. (2013) devised a second-order approximation of the gradient of log-likelihood using sequential Monte Carlo smoothing. In our work, we first introduce the generalization of Stein’s identity for normal distribution to p-generalized Gaussian distribution, enabling more flexible perturbation with different tail behaviors. Building upon these gradient approximations, we introduce a novel weighted average algorithm for maximizing likelihood through the two-time-scale stochastic approximation. We show the integration of the algorithm into iterated filtering framework, relaxing the requirement for a bounded variance of the two-timescale stochastic approximation. Initially, we apply p-generalised Gaussian smoothing through Weighted Average Iterated filtering in two toy problems: a linear ou2 model and a nonlinear Gompertz model. Subsequently, we demonstrate the potential of this technique in f itting a more complex cholera model, incorporating a highly nonlinear structure with discrete population dynamics, seasonality, and extra-demographic stochasticity.
References
Arnaud Doucet, Pierre E Jacob, and Sylvain Rubenthaler. Derivative-free estimation of the score vector and observed information matrix with application to state-space models. arXiv preprint arXiv:1304.5768, 2013.
Edward L Ionides, Carles Bret´ o, and Aaron A King. Inference for nonlinear dynamical systems. Proceedings of the National Academy of Sciences, 103(49):18438–18443, 2006.
Relational Format
conference proceeding
Recommended Citation
Qazi, Zamzam, "Weighted Average Iterated filtering using p-generalized Gaussian smoothing" (2024). AMS-AWM Student Research Symposium. 13.
https://egrove.olemiss.edu/ams_awm_sympo/2024/schedule/13
Weighted Average Iterated filtering using p-generalized Gaussian smoothing
Room 321, Hume Hall
In recent years, simulation-based inferences have garnered significant attention due to the inherent challenges in directly computing likelihood functions for many real-world problems. Iterated f iltering Ionides et al. (2006) has emerged as a method to maximize likelihood functions by perturbing models and approximating the gradient of log-likelihood through sequential Monte Carlo f iltering. Using Stein’s identity, Doucet et al. (2013) devised a second-order approximation of the gradient of log-likelihood using sequential Monte Carlo smoothing. In our work, we first introduce the generalization of Stein’s identity for normal distribution to p-generalized Gaussian distribution, enabling more flexible perturbation with different tail behaviors. Building upon these gradient approximations, we introduce a novel weighted average algorithm for maximizing likelihood through the two-time-scale stochastic approximation. We show the integration of the algorithm into iterated filtering framework, relaxing the requirement for a bounded variance of the two-timescale stochastic approximation. Initially, we apply p-generalised Gaussian smoothing through Weighted Average Iterated filtering in two toy problems: a linear ou2 model and a nonlinear Gompertz model. Subsequently, we demonstrate the potential of this technique in f itting a more complex cholera model, incorporating a highly nonlinear structure with discrete population dynamics, seasonality, and extra-demographic stochasticity.
References
Arnaud Doucet, Pierre E Jacob, and Sylvain Rubenthaler. Derivative-free estimation of the score vector and observed information matrix with application to state-space models. arXiv preprint arXiv:1304.5768, 2013.
Edward L Ionides, Carles Bret´ o, and Aaron A King. Inference for nonlinear dynamical systems. Proceedings of the National Academy of Sciences, 103(49):18438–18443, 2006.
Comments
poster