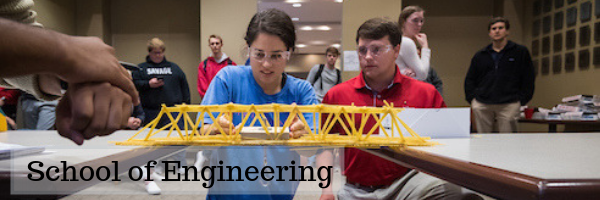
Faculty and Student Publications
Document Type
Article
Publication Date
5-2-2020
Abstract
© 2020 by the authors. Licensee MDPI, Basel, Switzerland. Virtual reality (VR) has advanced rapidly and is used for many entertainment and business purposes. The need for secure, transparent and non-intrusive identification mechanisms is important to facilitate users’ safe participation and secure experience. People are kinesiologically unique, having individual behavioral and movement characteristics, which can be leveraged and used in security sensitive VR applications to compensate for users’ inability to detect potential observational attackers in the physical world. Additionally, such method of identification using a user’s kinesiological data is valuable in common scenarios where multiple users simultaneously participate in a VR environment. In this paper, we present a user study (n = 15) where our participants performed a series of controlled tasks that require physical movements (such as grabbing, rotating and dropping) that could be decomposed into unique kinesiological patterns while we monitored and captured their hand, head and eye gaze data within the VR environment. We present an analysis of the data and show that these data can be used as a biometric discriminant of high confidence using machine learning classification methods such as kNN or SVM, thereby adding a layer of security in terms of identification or dynamically adapting the VR environment to the users’ preferences. We also performed a whitebox penetration testing with 12 attackers, some of whom were physically similar to the participants. We could obtain an average identification confidence value of 0.98 from the actual participants’ test data after the initial study and also a trained model classification accuracy of 98.6%. Penetration testing indicated all attackers resulted in confidence values of less than 50% (<50%), although physically similar attackers had higher confidence values. These findings can help the design and development of secure VR systems.
Relational Format
journal article
Recommended Citation
Olade, I., Fleming, C., & Liang, H.-N. (2020). BioMove: Biometric User Identification from Human Kinesiological Movements for Virtual Reality Systems. Sensors, 20(10), 2944. https://doi.org/10.3390/s20102944
DOI
10.3390/s20102944
Accessibility Status
Searchable text