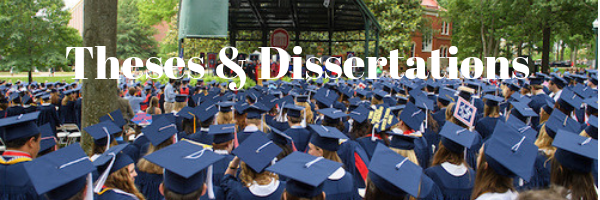
Date of Award
1-1-2015
Document Type
Dissertation
Degree Name
Ph.D. in Mathematics
Department
Mathematics
First Advisor
Xin Dang
Second Advisor
John P. Bentley
Third Advisor
Hailin Sang
Relational Format
dissertation/thesis
Abstract
Gini's mean difference (GMD) and its derivatives such as Gini index have been widely used as alternative measures of variability over one century in many research fields especially in finance, economics and social welfare. In this dissertation, we generalize the univariate GMD to the multivariate case and propose a new covariance matrix so called the Gini covariance matrix (GCM). The extension is natural, which is based on the covariance representation of GMD with the notion of multivariate spatial rank function. In order to gain the affine equivariance property for GCM, we utilize the transformation-retransformation (TR) technique and obtain TR version GCM that turns out to be a symmetrized M-functional. Indeed, both GCMs are symmetrized approaches based on the difference of two independent variables without reference of a location, hence avoiding some arbitrary definition of location for non-symmetric distributions. We study the properties of both GCMs. They possess the so-called independence property, which is highly important, for example, in independent component analysis. Influence functions of two GCMs are derived to assess their robustness. They are found to be more robust than the regular covariance matrix but less robust than Tyler and Dümbgen M-functional. Under elliptical distributions, the relationship between the scatter parameter and the two GCM are obtained. With this relationship, principal component analysis (PCA) based on GCM is possible.
Estimation of two GCMs is presented. We study asymptotical behavior of the estimators. √n-consistency and asymptotical normality of estimators are established. Asymptotic relative efficiency (ARE) of TR-GCM estimator with respect to sample covariance matrix is compared to that of Tyler and Dümbgen M-estimators. With little loss on efficiency (< 2%) in the normal case, it gains high efficiency for heavy-tailed distributions. Finite sample behavior of Gini estimators is explored under various models using two criteria. As a by-product, a closely related scatter Kotz functional and its estimator are also studied.
The proposed Gini covariance balances well between efficiency and robustness. In applications, we implement the Gini-based PCA to two real data sets from UCI machine learning repository. Relying on some graphical and numerical summaries, Gini-based PCA demonstrates its competitive performance.
Recommended Citation
Weatherall, Lauren Anne, "Gini Covariance Matrix and its Affine Equivariant Version" (2015). Electronic Theses and Dissertations. 1441.
https://egrove.olemiss.edu/etd/1441