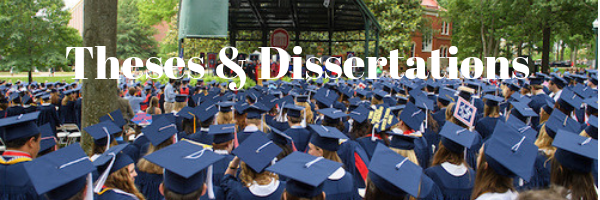
Date of Award
2019
Document Type
Dissertation
Degree Name
Ph.D. in Engineering Science
Department
Electrical Engineering
First Advisor
Paul Goggans
Second Advisor
Lei Cao
Third Advisor
Yixin Chen
Relational Format
dissertation/thesis
Abstract
Bayesian model comparison provides a rational and consistent method for applying logic and probability to the problem of evaluating models. Model comparison requires numerical techniques that are usually very time consuming to run. This dissertation proposes extensions to several existing numerical model comparison techniques, including nested sampling and thermodynamic integration, that incorporate parallel algorithm design to achieve significant speed-ups. Serial computer performance gains have sloin recent years, and most processing speed improvements are seen in the area of parallel architectures. This work discusses the design, theoretical analysis, and empirical analysis of these algorithms, focusing on the performance of these algorithms with respect to accuracy and run time. Many disciplines in science and engineering make use of existing model comparison techniques. This work aims to save investigators in these disciplines time, and potentially attract those who may have been put off by time complexity concerns, by developing a general approach to model comparison that takes full advantage of modern parallel computing platforms.
Recommended Citation
Henderson, Robert Wesley, "Design and Analysis of Efficient Parallel Bayesian Model Comparison Algorithms" (2019). Electronic Theses and Dissertations. 1694.
https://egrove.olemiss.edu/etd/1694