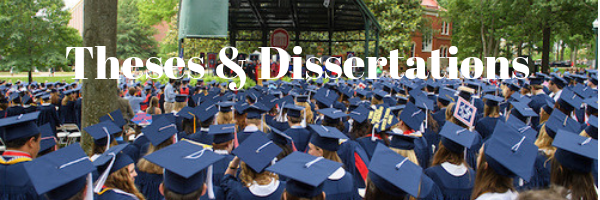
Date of Award
1-1-2021
Document Type
Dissertation
Degree Name
Ph.D. in Engineering Science
First Advisor
Yacoub M. YMN Najjar
Second Advisor
Hakan HY Yasarer
Third Advisor
Yacoub YMN Najjar
Relational Format
dissertation/thesis
Abstract
An efficient and safe road network secures the nation’s economy and prosperity by providing public mobility and freight transport. Maintenance and rehabilitation of the road network cost billions of dollars annually. Road and highway infrastructures performance in any country is impacted by load repetitions and it is further compromised by climate attributes and extreme weather events. Damages to roads and bridges are among the infrastructure failures that have occurred during these extreme events. If maintenance and rehabilitation are not done promptly, the damages to the road caused by heavy traffic and extreme climate may lead to life-threatening conditions for road users. A disruption in any one system affects the performance of others. For example, damages in road and bridge infrastructure will delay the recovery operation after a disaster. In 2018, a total of 331 natural disaster occurrences were reported worldwide, which resulted in 14,385 deaths. From 1900 to 2000, in 119 years, 14,854 natural disaster occurrences were reported which caused 32,651,605 deaths. Natural disaster occurrences like hurricanes, floods, droughts, landslides, etc. may be influenced by specific climate mechanisms like El Niño and Southern Oscillation (ENSO). Several climate attributes models were developed in this research employing Auto-Regressive Integrated Moving Average (ARIMA) methodology. The sea surface temperature data were analyzed and a prediction model was developed to predict future ENSO years. The model successfully predicted the 2018-2019 El Niño year. The model prediction showed that the next El Niño years will be 2021-22 and 2025-26. The model prediction also shows that the next La Niña year will be 2028-29. Global mean sea level (GMSL) data were analyzed and a prediction model was developed. The predicted annual rate of change in GMSL is 0.6 mm/year from 2013 to 2050. But a higher annual rate of change (1.4 mm/year) is predicted from 2031 to 2050. Northern hemisphere (Arctic) sea ice extent and southern hemisphere (Antarctic) sea ice extent data were investigated and two different models were developed. The model prediction shows that the total loss of northern hemisphere sea ice extent in 2050 will be 1.66 million km2. But the total gain of southern hemisphere sea ice extent will be 1.24 million km2. The net change of global sea ice extent will be -0.24 million km2, which indicates a loss of sea ice. The model predictions of the climate attributes can be used to understand and assess the future climate change in different climate zones worldwide. This understanding of climate changes and future predictions of climate attributes will help to develop climate adaptation strategies and better prepare the communities for extreme weather-related natural disaster occurrences.
The condition deterioration progression of infrastructures, such as roads and bridges, is caused by load repetitions, as well as climate attributes and extreme weather. Pavements undergo maintenance and rehabilitations periodically to provide a smooth riding experience to the riders. Previous researches never considered maintenance and rehabilitation action history in the development of the condition deterioration model. This research considered the maintenance and rehabilitation history in the development and implementation of pavement condition deterioration models. The development of the IRI prediction model using Artificial Neural Network (ANN) and Multiple Linear Regression (MLR) considered the Long Term Pavement Performance (LTPP) climatic region, pavement structural properties, and traffic. The developed models are more objective, incorporate important input variables that are easily available, and are easy to implement in decision making. The concrete highway pavement IRI deterioration prediction models were developed and evaluated in this research for LTPP datasets of 1,482 for JPCP, 577 for JRCP, and 575 for CRCP. Comparatively, the AASHTO MEPDG performance equations were developed using fewer test sections.
Three performance models were developed for output variable, IRI (outside wheel path) (m/km) for Jointed Plain Concrete Pavement (JPCP), Jointed Reinforced Concrete Pavement (JRCP), and Continuously Reinforced Concrete Pavement (CRCP). The input variables are similar for all the models. An in-depth study of M&R history collected from the LTPP database for all concrete pavement produced several CN_Code. The best models were found with the CN_Code developed based on the IRI value improvement and the type of M&R action and this variable is a continuous variable where number increment indicates the frequency of M&R action provided in the pavement section.
The models’ final structure and accuracy statistics can be summarized as: JPCP (13-19-1; ANN R2 =0.94 and MLR R2 =0.49), JRCP (11-19-1; ANN R2 =0.95 and MLR R2 =0.58), and CRCP (14-19-1; ANN R2 =0.95 and MLR R2 =0.83). The ANN models show better accuracy in predicting pavement performance compare to the multiple regression models for all types of concrete pavements. The developed IRI prediction models can successfully characterize the behavior (i.e. the increase of IRI values with time and decrease of IRI value after maintenance and rehabilitation). The ANN models can be used to provide future M&R action by changing CN_Code frequency and the model successfully distinguishes the behavior of IRI (i.e. decrease of IRI after M&R action and increase of IRI with time as CESAL increases). The developed condition deterioration models for concrete highway pavement present a significant improvement on the models currently used in the mechanistic-empirical pavement design method. It is recommended to implement the pavement condition deterioration model developed in this research for life-cycle asset management and M&R programs.
Recommended Citation
Sultana, Salma, "COMPUTATIONAL MODELING OF CLIMATE ATTRIBUTES AND CONDITION DETERIORATION OF CONCRETE HIGHWAY PAVEMENTS" (2021). Electronic Theses and Dissertations. 2138.
https://egrove.olemiss.edu/etd/2138