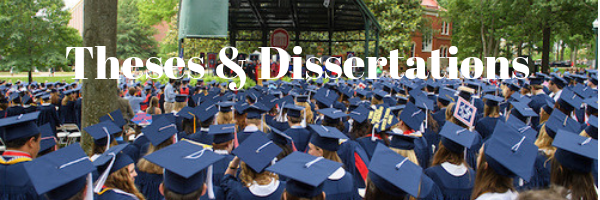
Date of Award
2013
Document Type
Dissertation
Degree Name
Ph.D. in Engineering Science
Department
Geology and Geological Engineering
First Advisor
Greg Easson
Second Advisor
Jay K. Johnson
Third Advisor
Clifford A. Ochs
Relational Format
dissertation/thesis
Abstract
Lakes and reservoirs are important resources that provide water for critical needs, such as drinking water, agriculture, recreation, fisheries, wildlife, and other uses. However, there is increasing concern that anthropogenic eutrophication threatens the usability of these natural resources. Therefore, this research investigates these complex hydrologic ecosystems and recommends a methodology for monitoring the trophic state of lakes and reservoirs using remote sensing data. The Mississippi Department of Environmental Quality provided in situ data for seven Mississippi lakes including, Arkabutla, Bay Springs, Enid, Grenada, Okatibbee, Ross Barnett, and Sardis lakes. This research explored the relationships between the Secchi depth (SD), chlorophyll-a (CHL), and total phosphorus (TP) in situ data and Moderate Resolution Imaging Spectroradiometer (MODIS) spectral reflectance data. This was accomplished by deriving Carlson Trophic State Index values for each in situ measurements and using these TSI(SD), TSI(CHL), and TSI(TP) values to evaluate potential predictive methods. Simple linear regression was performed to quantify the strength of the relationships between the in situ data and MODIS surface reflectance values. However, R-square values were too low and inconsistent to justify additional analyses. Therefore, machine learning models from the Waikato Environment for Knowledge Analysis (WEKA) software workbench were explored and tested. Optimal predictive models and settings were investigated for two meta-learner classifiers, three Bayesian classifiers and three decision tree classifiers. The Classification Via Regression yielded the best results when using large datasets, the all-but-one iteration setting, MODIS A1 individual bands as predictors, and TSI(SD) as targets. For this model and these settings, the percentages of correctly classified instances ranged from 77.74% to 81.98% and kappa values ranged from 0.41 to 0.48. The percentage of correctly classified results by class class for TSI(SD) were 39.80% for hyperturbidity and 85.11% for turbidity. Overall, this research concludes that Moderate Resolution Imaging Spectroradiometer (MODIS) satellite imagery can be used to effectively monitor Mississippi lakes and reservoirs. Additionally, machine learning models were determined to be a viable option for predicting water transparency measurements. It is anticipated that water resource managers can adopt these research findings to complement conventional in situ lake monitoring methods.
Recommended Citation
Aten, Michelle, "Trophic State Monitoring Of Lakes And Reservoirs Using Remote Sensing" (2013). Electronic Theses and Dissertations. 607.
https://egrove.olemiss.edu/etd/607
Concentration/Emphasis
Emphasis: Geology