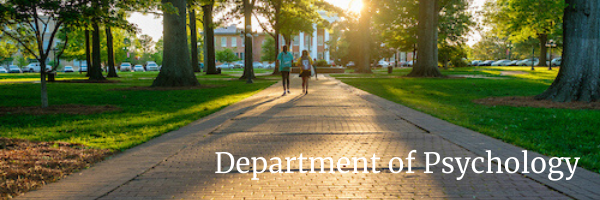
Faculty and Student Publications
Document Type
Article
Publication Date
7-15-2019
Abstract
© 2019, The Psychonomic Society, Inc. Maximum-likelihood estimation of the parameters of a psychometric function typically occurs through an iterative search for the maximum value in the likelihood function defined across the parameter space. This procedure is subject to failure. First, iterative search procedures may converge on a local, not global, maximum in the likelihood function. The procedure also fails when the likelihood function does not contain a maximum. This is the case when either a step function or a constant function is associated with a higher likelihood than the model can attain with finite parameter values. In such cases iterative search procedures may erroneously report having successfully converged on a maximum in the likelihood function. This will lead not only to inaccurate models for the observed data, but may also lead to inaccurate results regarding the reliability of parameter estimates, goodness-of-fit of the model, or model selection. I describe a method by which such false convergences can be reliably detected. I also present results of simulations that systematically investigate how stimulus placement, number of trials, parameters estimated, task (2AFC, 4AFC, etc.), and whether the lapse rate is allowed to vary affect the probability that the likelihood function will not contain a maximum. Based on the results of the simulations recommendations are made regarding experimental design and modeling choices. Software that implements the method is made available for downloading.
Relational Format
journal article
Recommended Citation
Prins, N. (2019). Too much model, too little data: How a maximum-likelihood fit of a psychometric function may fail, and how to detect and avoid this. Attention, Perception, & Psychophysics, 81(5), 1725–1739. https://doi.org/10.3758/s13414-019-01706-7
DOI
10.3758/s13414-019-01706-7
Accessibility Status
Searchable text