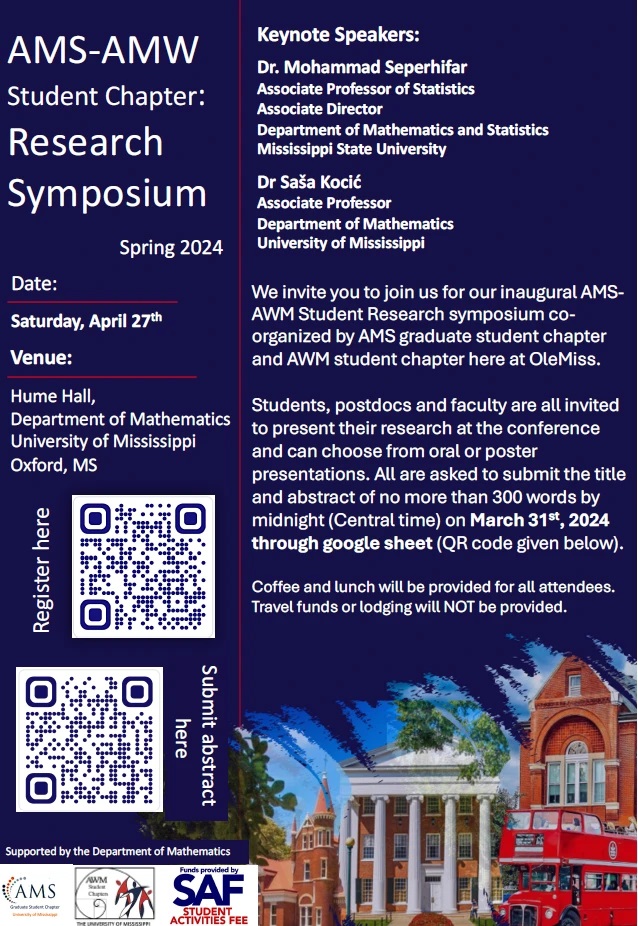
Exploring Economic Scenario Generator in Central Africa: A Focus on Time Series and Copulas
Location
Room 321, Hume Hall
Start Date
27-4-2024 10:00 AM
End Date
27-4-2024 11:00 AM
Description
Employing six variables, encompassing three macroeconomic indicators for Cameroon and three financial variables from BVMAC (the Central Africa Stock Exchange), we present a customized Economic Scenario Generator (ESG) designed for Central African countries to enhance risk management in the economic sector. Our approach involves a time series analysis (ARIMA models) of your variables and an analysis of residuals generated by these models. Faced with the inadequacy of correlation coefficients in the context of non-elliptical distributions of residuals for some variables, we study their dependencies using copulas. Based on the results of this dependency, we use Cholesky decomposition (specific to elliptical distributions) to project macroeconomic variables. Aware of the difficulty in projecting all our variables, especially those whose residuals do not follow a normal distribution, we pose an open problem in this regard. The dataset is divided into training and test sets, thereby reinforcing the effectiveness and reliability of the ESG.
Relational Format
conference proceeding
Recommended Citation
Longla, Martial; Imandi, Régine Constella; and Fono, Louis Aimé, "Exploring Economic Scenario Generator in Central Africa: A Focus on Time Series and Copulas" (2024). AMS-AWM Student Research Symposium. 8.
https://egrove.olemiss.edu/ams_awm_sympo/2024/schedule/8
Exploring Economic Scenario Generator in Central Africa: A Focus on Time Series and Copulas
Room 321, Hume Hall
Employing six variables, encompassing three macroeconomic indicators for Cameroon and three financial variables from BVMAC (the Central Africa Stock Exchange), we present a customized Economic Scenario Generator (ESG) designed for Central African countries to enhance risk management in the economic sector. Our approach involves a time series analysis (ARIMA models) of your variables and an analysis of residuals generated by these models. Faced with the inadequacy of correlation coefficients in the context of non-elliptical distributions of residuals for some variables, we study their dependencies using copulas. Based on the results of this dependency, we use Cholesky decomposition (specific to elliptical distributions) to project macroeconomic variables. Aware of the difficulty in projecting all our variables, especially those whose residuals do not follow a normal distribution, we pose an open problem in this regard. The dataset is divided into training and test sets, thereby reinforcing the effectiveness and reliability of the ESG.
Comments
poster