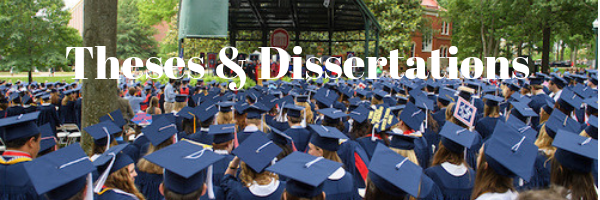
Date of Award
1-1-2012
Document Type
Dissertation
Degree Name
Ph.D. in Engineering Science
Department
Computer and Information Science
First Advisor
Yixin Chen
Second Advisor
Scott A. Gustafson
Third Advisor
Dwight E. Waddell
Relational Format
dissertation/thesis
Abstract
A Brain-Computer Interface (BCI) provides a bidirectional communication path for a human to control an external device using brain signals. Among neurophysiological features in BCI systems, steady state visually evoked potentials (SSVEP), natural responses to visual stimulation at specific frequencies, has increasingly drawn attentions because of its high temporal resolution and minimal user training, which are two important parameters in evaluating a BCI system. The performance of a BCI can be improved by a properly selected neurophysiological signal, or by the introduction of machine learning techniques. With the help of machine learning methods, a BCI system can adapt to the user automatically.
In this work, a machine learning approach is introduced to the design of an SSVEP based BCI. The following open problems have been explored:
1. Finding a waveform with high success rate of eliciting SSVEP. SSVEP belongs to the evoked potentials, which require stimulations. By comparing square wave, triangle wave and sine wave light signals and their corresponding SSVEP, it was observed that square waves with 50% duty cycle have a significantly higher success rate of eliciting SSVEPs than either sine or triangle stimuli.
2. The resolution of dual stimuli that elicits consistent SSVEP. Previous studies show that the frequency bandwidth of an SSVEP stimulus is limited. Hence it affects the performance of the whole system. A dual-stimulus, the overlay of two distinctive single frequency stimuli, can potentially expand the number of valid SSVEP stimuli. However, the improvement depends on the resolution of the dual stimuli. Our experimental results shothat 4 Hz is the minimum difference between two frequencies in a dual-stimulus that elicits consistent SSVEP.
3. Stimuli and color-space decomposition. It is known in the literature that although low-frequency stimuli (<30 Hz) elicit strong SSVEP, they may cause dizziness. In this work, we explored the design of a visually friendly stimulus from the perspective of color-space decomposition. In particular, a stimulus was designed with a fixed luminance component and variations in the other two dimensions in the HSL (Hue, Saturation, Luminance) color-space. Our results shothat the change of color alone evokes SSVEP, and the embedded frequencies in stimuli affect the harmonics. Also, subjects claimed that a fixed luminance eases the feeling of dizziness caused by low frequency flashing objects.
4. Machine learning techniques have been applied to make a BCI adaptive to individuals. An SSVEP-based BCI brings new requirements to machine learning. Because of the non-stationarity of the brain signal, a classifier should adapt to the time-varying statistical characters of a single user's brain wave in realtime. In this work, the potential function classifier is proposed to address this requirement, and achieves 38.2bits/min on offline EEG data.
Recommended Citation
Teng, Fei, "An SSVEP Brain-Computer Interface: A Machine Learning Approach" (2012). Electronic Theses and Dissertations. 1374.
https://egrove.olemiss.edu/etd/1374