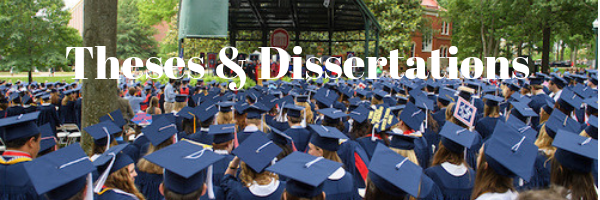
Date of Award
1-1-2012
Document Type
Dissertation
Degree Name
Ph.D. in Mathematics
Department
Mathematics
First Advisor
Xin Dang
Second Advisor
Yixin Chen
Third Advisor
Ali Al-Sharadqah
Relational Format
dissertation/thesis
Abstract
Classical multivariate statistical inference methods including multivariate analysis of variance, principal component analysis, factor analysis, canonical correlation analysis are based on sample covariance matrix. Those moment-based techniques are optimal (most efficient) under the normality distributional assumption. They are, however, extremely sensitive to outlying observations, susceptible to small perturbation in data and poor in the efficiency for heavy-tailed distributions. A straightforward treatment is to replace the sample covariance matrix with a robust one. Visuri et al. (2000) proposed a technique for robust covariance matrix estimation based on different notions of multivariate sign and rank. Among them, the spatial rank based covariance matrix estimator that utilizes a robust scale estimator (MRCM) is especially appealing due to its high robustness, computational ease and good efficiency. In this dissertation, properties of the estimator on orthogonal equivariance under any distribution and affine equivariance under elliptically symmetric distributions have been established. The major robustness properties of the estimator are studied by the breakdown point and influence function analysis. More specifically, the finite sample breakdown point is obtained and the upper bound of the finite sample breakdown point can be achieved by a proper choice of univariate robust scale estimator. The influence functions for eigenvalues and eigenvectors of the estimator are derived. They are found to be bounded under some mild assumptions. Moreover, empirical comparisons to popular robust MCD, M and S estimators show that MRCM has a competitive performance on efficiency as well as robustness. With rapid advances in information technology, data have been becoming huge in size and complex in structure. A single elliptical distribution is no longer sufficient to model such data. This motivates a generalization of our notion of MRCM to mixture models. In this dissertation, we propose a robust Spatial-EM algorithm for estimating parameters in the mixture model. Rather than using sample covariance matrix in each M-step, Spatial-EM ingeniously implements MRCM to enhance stability and robustness of the estimation procedure. Analyzing the log-likelihood function, the proposed one is found to be closely related to the maximum likelihood estimator (MLE) of Kotz type mixture model. Comparing with the direct MLE, Spatial-EM has advantages in computation ease as well as stability. Applications of Spatial-EM to data mining become natural. We illustrate procedures how to use Spatial-EM for supervised and unsupervised learning problems. More specifically, robust clustering and outlier detection methods based on Spatial-EM have been proposed. We adopt the outlier detection to taxonomic research on fish species novelty discovery. UCI Wisconsin diagnostic breast cancer data and Yeast cell cycle data are used for clustering analysis. Comparing with the regular EM and many other existing methods such as X-EM and SVM, Spatial-EM demonstrates its competitive classification power and high robustness.
Classical multivariate statistical inference methods including multivariate analysis of variance, principal component analysis, factor analysis, canonical correlation analysis are based on sample covariance matrix. Those moment-based techniques are optimal (most efficient) under the normality distributional assumption. They are, however, extremely sensitive to outlying observations, susceptible to small perturbation in data and poor in the efficiency for heavy-tailed distributions. A straightforward treatment is to replace the sample covariance matrix with a robust one. Visuri et al. (2000) proposed a technique for robust covariance matrix estimation based on different notions of multivariate sign and rank. Among them, the spatial rank based covariance matrix estimator that utilizes a robust scale estimator (MRCM) is especially appealing due to its high robustness, computational ease and good efficiency. In this dissertation, properties of the estimator on orthogonal equivariance under any distribution and affine equivariance under elliptically symmetric distributions have been established. The major robustness properties of the estimator are studied by the breakdown point and influence function analysis. More specifically, the finite sample breakdown point is obtained and the upper bound of the finite sample breakdown point can be achieved by a proper choice of univariate robust scale estimator. The influence functions for eigenvalues and eigenvectors of the estimator are derived. They are found to be bounded under some mild assumptions. Moreover, empirical comparisons to popular robust MCD, M and S estimators show that MRCM has a competitive performance on efficiency as well as robustness.
With rapid advances in information technology, data have been becoming huge in size and complex in structure. A single elliptical distribution is no longer sufficient to model such data. This motivates a generalization of our notion of MRCM to mixture models. In this dissertation, we propose a robust Spatial-EM algorithm for estimating parameters in the mixture model. Rather than using sample covariance matrix in each M-step, Spatial-EM ingeniously implements MRCM to enhance stability and robustness of the estimation procedure. Analyzing the log-likelihood function, the proposed one is found to be closely related to the maximum likelihood estimator (MLE) of Kotz type mixture model. Comparing with the direct MLE, Spatial-EM has advantages in computation ease as well as stability.
Applications of Spatial-EM to data mining become natural. We illustrate procedures how to use Spatial-EM for supervised and unsupervised learning problems. More specifically, robust clustering and outlier detection methods based on Spatial-EM have been proposed. We adopt the outlier detection to taxonomic research on fish species novelty discovery. UCI Wisconsin diagnostic breast cancer data and Yeast cell cycle data are used for clustering analysis. Comparing with the regular EM and many other existing methods such as X-EM and SVM, Spatial-EM demonstrates its competitive classification power and high robustness.
Recommended Citation
Yu, Kai, "Contributions to Robust Methods: Modified Rank Covariance Matrix and Spatial-EM Algorithm" (2012). Electronic Theses and Dissertations. 1438.
https://egrove.olemiss.edu/etd/1438