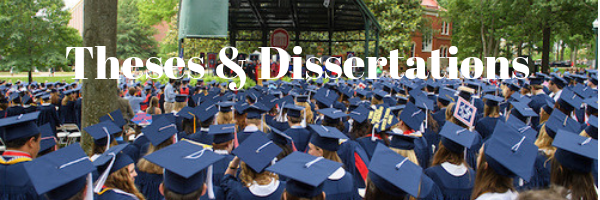
Date of Award
1-1-2014
Document Type
Dissertation
Degree Name
Ph.D. in Mathematics
Department
Mathematics
First Advisor
Xin Dang
Second Advisor
Walter Mayer
Third Advisor
Gerard Buskes
Relational Format
dissertation/thesis
Abstract
The problem of testing whether two samples come from the same or different population is a classical one in statistics. In this dissertation, I first study rank based formulation of univariate two-sample distribution-free tests. One form of the test statistic is the average of between-group distances of ranks. The other form of the test statistic is the difference between the average of between-group distances of ranks and the average of within-group distances of ranks. Although they are different in formulation, they are closely related to the two-sample Cramer-von Mises criterion. The first one is a linear transformation of Cramer-von Mises criterion in the case the two samples are of the same size. The second one is a different form of the Cramer-von Mises criterion. The properties of the two-sample test statistic based on the new formulation are studied. In particular, the Hajek projection and orthogonal decomposition technique in deriving the asymptotics of the test statistic is applied. For the first statistic under the balanced case, its limiting distribution is not normal since the projection on one variable is insufficient to represent the variation of the test statistic. By taking the projection on two variables, it is proved to be a weighted mixture of independent chi-square distributions. An operator in the functional space is defined and its eigenfunctions and eigenvalues are applied to derive the limiting distribution. Rank-based formulations allow generalizations of the two-sample Cramer-von Mises test to the multivariate case by using different notions of multivariate rank functions. In the multivariate case, the rank tests may lose the distribution-free property under a general alternative. They are, however, usually more robust than the parametric tests. I propose two corresponding new tests based on multivariate spatial ranks. The spatial rank function yields a relative center-outward ranking of a data set. It preserves not only ordering on the magnitude of vectors but also directional information. It characterizes the distribution. One test statistic is the difference between the average of intra-sample rank distances and the average of inter-sample rank distances. The other one is simply the average of intra-sample rank distances for the balanced samples. Unlike the univariate case, those two statistics are no longer equivalent. Comparing with other tests, the proposed ones can be established by the following desirable properties. (1) They are nonparametric with fewer assumptions, although they are not completely distribution-free. (2) They are invariant with respect to orthogonal linear transformations, which doesn't hold for tests based on the component-wise ranks. (3) They are consistent against all alternatives. The simulation results have illustrated the proposed tests to be promising. The bootstrap and permutation procedures are used for yielding a consistent approximation to the null distribution of the test statistics.
Recommended Citation
Curry, Jamye, "Rank-based Two Sample Tests Under a General Alternative" (2014). Electronic Theses and Dissertations. 1440.
https://egrove.olemiss.edu/etd/1440