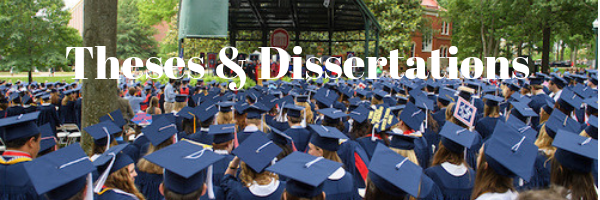
Date of Award
1-1-2021
Document Type
Dissertation
Degree Name
Ph.D. in Mathematics
Department
Mathematics
First Advisor
Dao D. Nguyen
Second Advisor
Xin X. Dang
Third Advisor
Hailin H. Sang
Relational Format
dissertation/thesis
Abstract
In this dissertation, We show the results of our researches in statistical sampling, functional optimization, and methodology for partially observed Markov process (POMP) models. In statistical sampling, we introduce a p-generalized smoothing method that enables the Langevin-Monte Carlo method to generate a sample from a log concave distribution weakly smoothing potential function. For our optimization research, we introduce an accelerated inexact gradient (AIG) method. Combining the strengths while mitigating the weakness of its parent methods: gradient descent and Nesterov's accelerated gradient, AIG converges with excellent rates for both convex and non-convex optimization problems for smooth objective functions. Furthermore, we also adapt AIG into iterated filtering (IF), which is a parameter estimation method on the partially observed Markov process models. This vastly improves both the accuracy and precision of IF, allowing it to perform favorably compared to the state of the art methods in our simulation example. Finally, we introduce our R package "is2", which extends some useful Monte Carlo statistical methodologies to improve on convergence rates and inherits the flexible framework of R package "pomp". A variety of efficient statistical methods for POMP models have been developed including fixed lag smoothing, second-order iterated smoothing, momentum iterated filtering, average iterated filtering, accelerated iterated filtering and particle iterated filtering. In this dissertation, we show the utility of these methodologies using two toy problems. We also demonstrate the applicability of our methods on a more complex model, employing a nonlinear epidemiological model with a discrete population, seasonality, and extra-demographic stochasticity.
Recommended Citation
Doan, Duc Anh Anh, "TOPICS ON APPLICATIONS OF OPTIMIZATION THEORIES ON STATISTICAL METHODOLOGIES" (2021). Electronic Theses and Dissertations. 1997.
https://egrove.olemiss.edu/etd/1997