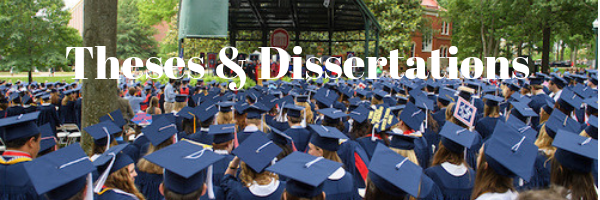
Date of Award
1-1-2021
Document Type
Thesis
Degree Name
M.S. in Engineering Science
First Advisor
Feng Wang
Second Advisor
Yixin Chen
Third Advisor
Dawn Wilkins
Relational Format
dissertation/thesis
Abstract
This thesis presents a novel approach towards high accuracy indoor localization with smartphones. Better than all the existing indoor localization methods, our approach has the advantage of being infrastructural-free, robust, and it does not require any pre-installation in a new environment. To make this goal come true, we built a testbed that only uses the Inertial Measurement Units (IMU) of the smartphones to access the smartphones' raw acceleration and orientation data, then use these data to calculate the user's location by coordinate transformation and interactions. We conducted extensive experiments and evaluations for testbed validation as well as to carefully examine in depth on the well-known drifting problem of the Inertial Navigation System (INS), which is caused by imprecise sensor readings. When walking straight, these sensor errors and noises show no regular patterns on the accelerations. However, after one-time integration on accelerations, the resulting velocities on each dimension show clear drifting patterns where they always drift in linear order, which is caused by the periodic drift in each step. Inspired by the drifting patterns, we found the drifting problem for walking in a straight line can be greatly reduced by performing linear regression on the velocities. As the normal pedestrian's walking behavior can be separated into walking straight section and turn section, we adopt a deep learning approach to treat them differently with different calibration techniques. Our calibrated result shows a great improvement comparing to the result calculated from the raw smartphone data.
Recommended Citation
Wang, Yunshu, "A Novel Approach Toward High Accuracy Indoor Localization}{Masters of Science" (2021). Electronic Theses and Dissertations. 2073.
https://egrove.olemiss.edu/etd/2073
Concentration/Emphasis
Computer Science