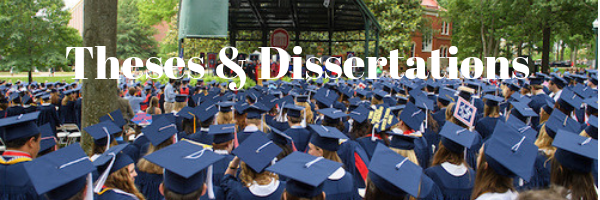
Date of Award
1-1-2021
Document Type
Dissertation
Degree Name
Ph.D. in Engineering Science
First Advisor
Dr. Hakan Yasarer
Second Advisor
Dr. Hunain Alkhateb
Third Advisor
Dr. Craig Hickey
Relational Format
dissertation/thesis
Abstract
Geotechnical measurements of soil parameters used in the design of infrastructure provide information at a specific point of the ground. The use of limited point data may result in greater uncertainty and less reliability in design. Geophysical methods are non-invasive, less time-consuming, and provide continuous spatial information about the soil. However, geophysical information is not in terms of engineering parameters. Correlations between geotechnical parameters and geophysical parameters are needed to facilitate the use of geophysical information in geotechnical designs. The current research is focused on two geophysical methods; electrical resistivity (ER) and seismic wave velocity (S-wave and P-wave). Artificial neural network (ANN) models are developed using published data to predict geotechnical parameters from ER and seismic wave velocity. Results of ANN models from the published data show that ER can predict geotechnical parameters with moderate to good accuracy and also predict cation exchange capacity (CEC) better than saturation. Seismic wave velocity helps to predict water content and dry density. Overall, the performance of ANN is better than regression. Laboratory measurements are performed on proctor-compacted soil samples with varying clay, sand, and silt proportions applicable to earthen dam construction. ER, seismic wave velocity and various geotechnical parameters are measured on the same samples. Results show that ER is most sensitive to Atterberg limits, specific surface area, CEC, cohesion, water content and saturation. ANN models are in agreement with the Waxman-Smits formula. In comparison to ER, S-wave and P-wave velocities are more sensitive to dry density and void ratio. Combining ER and S-wave and P-wave velocities predicts water content, dry density, saturation, and void ratio more accurately than simply using individual geophysical parameters. The geophysical parameters in conjunction with the soil mix proportions allow for good to high accuracy predictions of multiple geotechnical parameters.
Recommended Citation
Johora, Fatema Tuz, "FORECASTING GEOTECHNICAL PARAMETERS FROM ELECTRICAL RESISTIVITY AND SEISMIC WAVE VELOCITIES USING ARTIFICIAL NEURAL NETWORK MODELS" (2021). Electronic Theses and Dissertations. 2109.
https://egrove.olemiss.edu/etd/2109