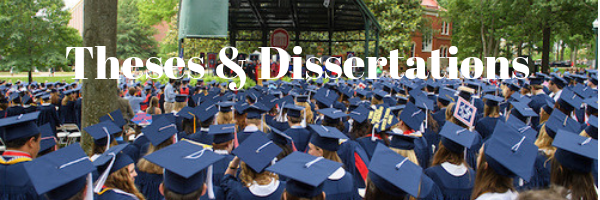
Date of Award
1-1-2022
Document Type
Dissertation
Degree Name
Ph.D. in Mathematics
Department
Mathematics
First Advisor
Xin Dang
Second Advisor
John Bentley
Third Advisor
Hailin Sang
Relational Format
dissertation/thesis
Abstract
The standard Gini correlation plays an important role in measuring the dependence between random variables with heavy-tailed distributions. It is based on the covariance between one variable and the rank of the other. Hence, for each pair of random variables, there are two Gini correlations and they are not equal in general, which brings a substantial difficulty in interpretation. We study two symmetric and computationally efficient Gini correlations with the computational complexity of O(nlogn). The properties of the two symmetric Gini correlations are explored. The influence function approach is utilized to study the robustness and the asymptotic behavior of these correlations. The asymptotic relative efficiencies and finite sample efficiencies are explored to compare these symmetric Gini correlations with several popular correlations under elliptical distributions as well as log-normal distributions. Most of the detailed work in this dissertation is directed toward the Pareto distribution due to its usefulness in modelling lifetime data, hydrology, competing risk data, and many other non-negative socio-economic issues. The Kendall tau and Gini correlations are explored in a bivariate Pareto distribution, comparing with the popular Pearson correlation and robust quadrant correlation. It is interesting to establish that a zero value in each of these correlations mutually imply independence in this family. The variance of the asymptotic normality for each sample correlation is derived via the influence function approach. When the second moment is finite, we demonstrate that the symmetric Gini correlation is asymptotically efficient as well as relatively efficient among finite samples. However, Kendall tau is more appealing in terms of compromising efficiency and robustness. Real data application is conducted to demonstrate the desirable performance of the two symmetric Gini correlations in this bivariate Pareto distribution.
Recommended Citation
Michael, Courtney Vanderford, "ON SYMMETRIC AND COMPUTATIONALLY EFFICIENT GINI CORRELATIONS IN SEVERAL BIVARIATE DISTRIBUTIONS DISSERTATION" (2022). Electronic Theses and Dissertations. 2256.
https://egrove.olemiss.edu/etd/2256