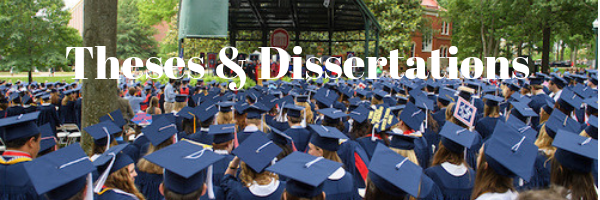
Date of Award
1-1-2023
Document Type
Dissertation
Degree Name
Ph.D. in Pharmaceutical Sciences
First Advisor
Sujith Ramachandran
Second Advisor
Yi Yang
Third Advisor
John P. Bentley
School
University of Mississippi
Relational Format
dissertation/thesis
Abstract
Background/Objectives
Adherence can impact the effectiveness of tumor necrosis factor inhibitors (TNFis) in treating rheumatoid arthritis. If rheumatoid arthritis reaches the end-stage, joint replacement may be needed. Unplanned readmissions after joint replacement is a Medicare quality measure. The first objective of this study was to determine the optimal adherence threshold for TNFis in rheumatoid arthritis. Using that threshold and the traditional 0.8 threshold, the second objective was to find the relationship between adherence to TNFis and opioid use in rheumatoid arthritis. Finally, prediction of unplanned readmissions in patients who had a knee or hip arthroplasty was the final objective.
Methods
A retrospective study design using a 5% sample of Medicare claims data from 2012-18 was used to answer the research questions. Survival analysis and time-dependent ROC curves were used to determine the optimal threshold of proportion of days covered (PDC) for TNFis. Panel analysis was performed to show the relationship between adherence and morphine milligram equivalents and length of opioid therapy. Machine learning algorithms were trained using the same predictors from the Medicare quality measure for classifying patients as having an unplanned readmission or not.
Results
Higher PDC was associated with lower risk of experiencing, inpatient admissions, oral glucocorticoid use, and a composite of all outcomes. The optimal preceding 90-day PDC threshold at 365 days was found to be 0.6. At the 0.6 and 0.8 PDC thresholds, adherence to TNFis was not significantly related to opioid use. In predicting readmissions after joint replacement, area under the receiver operating characteristic curve was poor, but the outcome was rare. Positive predictive value was highest in the random forest model at nearly 15%.
Implications/Conclusion
An optimal cut point backed by evidence can help ensure patients are being treated effectively and that research is conducted with more reliable cut points rather than an arbitrary cut point. However, the cut point found in study 1 does not relate to opioid use as was expected. Overall performance of the machine learning models was poor but could possibly be enhanced using electronic health record data or information from the initial admission to improve prediction. Accurate identification of patients who will be readmitted after joint replacement surgery can aid in measures to decrease the risk of readmission.
Recommended Citation
Toth Harris, Jennifer Marie, "Medication Use and Adherence in Rheumatoid Arthritis" (2023). Electronic Theses and Dissertations. 2591.
https://egrove.olemiss.edu/etd/2591