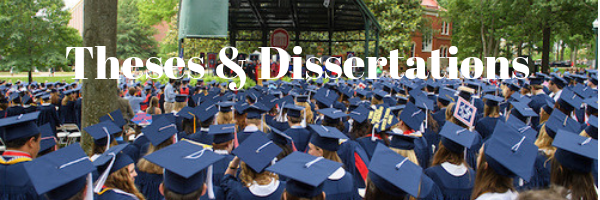
Date of Award
1-1-2023
Document Type
Thesis
Degree Name
M.S. in Engineering Science
First Advisor
Thai Le
Second Advisor
Charlie Walter
Third Advisor
Timothy Holston
School
University of Mississippi
Relational Format
dissertation/thesis
Abstract
LIME has emerged as one of the most commonly referenced tools in explainable AI (XAI) frameworks that is integrated into critical machine learning applications–e.g., healthcare and finance. However, its stability remains little explored, especially in the context of text data, due to the unique challenges when it comes to perturbing the underlying data, data that requires a stricter measure of semantic and syntactic consistency. To address these challenges, we first evaluate the inherent instability of LIME on text data to establish a baseline, and then propose a novel algorithm XAIFOOLER to perturb text inputs and manipulate explanations that casts investigation on the stability of LIME as a text perturbation optimization problem. XAIFOOLER conforms to the constraints to preserve text semantics and original model prediction with an enforcement of small, similar perturbations, and introduces Rank-biased Overlap (RBO) as a key part to guide the optimization of XAIFOOLER which is an alternative to more commonly used similarity measures that satisfies all the requirements necessary for an idea explanation similarity measure. Extensive experiments on real-world text datasets demonstrate that XAIFOOLER significantly outperforms all baselines by large margins in its ability to manipulate LIME’s explanations with high semantic preservability.
Recommended Citation
Burger, Christopher, "Adversarial Explanations for Text-Based Local Surrogate Models" (2023). Electronic Theses and Dissertations. 2665.
https://egrove.olemiss.edu/etd/2665