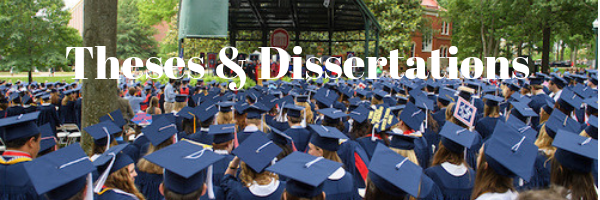
Date of Award
1-1-2023
Document Type
Dissertation
Degree Name
Ph.D. in Engineering Science
First Advisor
Ramanarayanan Viswanathan
Second Advisor
Md Sakib Hasan
Third Advisor
Kasem Khalil
School
University of Mississippi
Relational Format
dissertation/thesis
Abstract
In recent years, technological advancements in the field of computing have been limited by the slowing down of Moore’s law, which predicts the doubling of the number of transistors on a microchip every two years. The limitations of traditional solid-state electronics have become increasingly evident, as it is becoming difficult to increase the number of transistors while maintaining their reliability and performance. The breakdown of Dennard Scaling, which describes the power-density relationship in CMOS transistors, and the von Neumann bottleneck, which refers to the limited bandwidth between the central processing unit (CPU) and memory, have further exacerbated the situation. Moreover, the ever-increasing computing demands have led researchers to explore alternative computing mechanisms, such as neuromorphic computing.
In this context, there is a growing interest in developing novel memory devices that possess non-linear current-voltage characteristics and inherent memory properties, such as bio-inspired memory devices. These devices are inspired by the functioning of biological synapses in the brain, which enable neurons to communicate with each other and form complex networks. Bio-mem devices use biomolecules, such as proteins or DNA, as their active material and can exhibit a range of non-linear behaviors, including hysteresis, threshold switching, and negative differential resistance. Additionally, they have inherent memory properties that allow them to retain their state even after the power is turned off.
The unique features of bio-mem devices make them a promising candidate for solving classification and temporal pattern recognition tasks. Unlike their solid-state counterparts, bio-mem devices feature a similar structure, switching mechanism, and ionic transport modality as biological synapses, while consuming considerably lower power. The use of bio-mem devices in computing can lead to the development of more efficient and powerful computing systems that can handle complex tasks more effectively.
One promising computing paradigm that has emerged in recent years is Reservoir Computing (RC), which is a type of recurrent neural network that uses a fixed, random, and sparse connectivity matrix called a ”reservoir.” The reservoir provides a non-linear mapping of the input data, and the output is obtained by training a linear readout layer using a simple learning algorithm, such as ridge regression or support vector regression. RC has been shown to be effective in solving a range of classification and temporal problems, such as speech recognition, time-series prediction, and image classification. This work aims to explore the use of biomolecular devices in RC to solve classification and temporal problems, which could lead to the development of more efficient and powerful computing systems.
Recommended Citation
Hossain, Md Razuan, "Bio-Inspired Memory Device Based Physical Reservoir Computing System to Solve Temporal and Classification Problems" (2023). Electronic Theses and Dissertations. 2684.
https://egrove.olemiss.edu/etd/2684