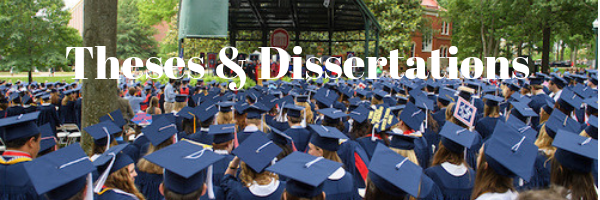
Date of Award
1-1-2023
Document Type
Dissertation
Degree Name
Ph.D. in Engineering Science
First Advisor
Charles Walter
Second Advisor
Charles Fleming
Third Advisor
Yixin Chen
Relational Format
dissertation/thesis
Abstract
In recent years the use of social media platforms, surveillance cameras, and vision-enabled devices and services has increased exponentially. While the mere use of a device or service is not a privacy issue, Machine Learning use has also drastically increased, and it exposes a number of privacy and security threats, especially towards online users. Privacy legislation around the world prohibits to use of data unfairly, but the conversion of laws to technical applications is often subject to interpretation. Such ambiguity opens a gap for data owners to potentially misuse data, violating end users’ privacy.
The overall objective of this doctoral research is to advance the state-of-the-art in privacy-preserving computer vision. Starting from work on privacy protection against intrusive facial recognition models, this research expands first into the realm of object detection, and then further to general Computer Vision tasks. A fast and effective adversarial algorithm is proposed against object detectors. By focusing on sensitive regions of images, this algorithm consistently beats state of the art systems. For general computer vision purposes, this research takes a task-agnostic approach by decoupling the privacy guarantee of data records from the tasks they are used for. This results in a tradeoff between image quality and privacy guarantees, which is measured empirically on example tasks. I discuss three examples of situations where privacy is not currently guaranteed, namely social media posts, surveillance cameras, and self-driving software, and show how each privacy-preserving tool can help mitigate the related residual privacy risk.
Recommended Citation
Cilloni, Thomas, "On Security and Privacy in Machine Learning" (2023). Electronic Theses and Dissertations. 2746.
https://egrove.olemiss.edu/etd/2746