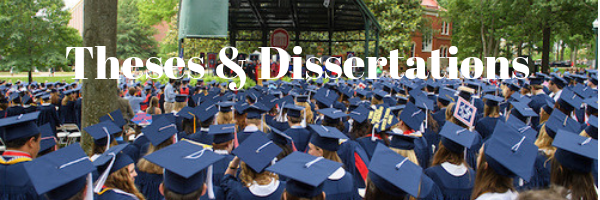
Date of Award
1-1-2024
Document Type
Dissertation
Degree Name
Ph.D. in Mathematics
First Advisor
Dr. Hailin Sang
Second Advisor
Dr. Xin Dang
Third Advisor
Dr. Jeremy Clark
Relational Format
dissertation/thesis
Abstract
In this dissertation, the focus is to investigate the convergence rates of error and estimation bounds of various f-divergence metrics in the context of generative adversarial networks (GANs). The error convergence rate is developed and explained by a class of functions with the discriminator and generator neural networks. The new class of functions is uniformly bounded, VC type, with bounded envelope function and Vapnik–Chervonenkis (VC) dimension dimension which makes it possible to apply Talagrand inequality. A tight convergence rate is derived for the error of GAN after applying the Talagrand inequality and Borel-Cantelli lemma. The error bound is generalized for the existing error estimation of GAN and we obtain a better convergence rate. We derive the convergence rate for the general objective function and the neural network distance which is a particular case of our technique. On the other hand, we aim to establish estimation bounds for various divergences, including total variation, Kullback-Leibler (KL) divergence, Hellinger divergence, and Pearson χ2 divergence, within the GAN estimator. We develop an inequality based on empirical and population objective functions, achieving almost surely convergence rates using Talagrand’s inequality. Subsequently, this inequality was employed to derive estimation bounds for total variation and KL divergence, leading to almost surely convergence rates and differences between the expected outputs of the discriminator on real data and generated data.
Recommended Citation
Hasan, Mahmud, "Error and f -Divergence Analysis of Generative Adversarial Network" (2024). Electronic Theses and Dissertations. 2815.
https://egrove.olemiss.edu/etd/2815