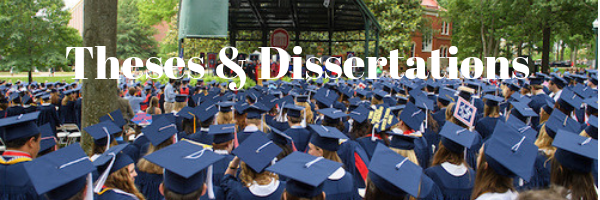
Date of Award
1-1-2024
Document Type
Thesis
Degree Name
M.S. in Engineering Science
First Advisor
John Daigle
Second Advisor
Lei Cao
Third Advisor
David Harrison
School
University of Mississippi
Relational Format
dissertation/thesis
Abstract
The objective of this thesis is to assess the performance of a data-drivennetwork scheduler in optimizing network performance in a three-slice 5G wireless cellular network. Each slice represents a distinct 5G traffic category or use case. These slices operate on the same underlying physical core and radio network; how- ever, they appear as independent networks from the end-user perspective. This work models the resource scheduling problem as a Markov decision process. A dataset of key performance measurements of a 5G network is constructed using MATLAB to train a deep Q-network reinforcement learning agent. Simulation results show that the agent can make scheduling decisions based on the performance of user endpoints (UEs). However, the reinforcement learning agent did not outperform the traditional round-robin scheduler in ensuring that all UEs achieve their performance objectives.
Recommended Citation
Omari, Mohammad, "A Machine Learning Approach to Managing 5G Network Slicing in the O-Ran Environment" (2024). Electronic Theses and Dissertations. 2966.
https://egrove.olemiss.edu/etd/2966