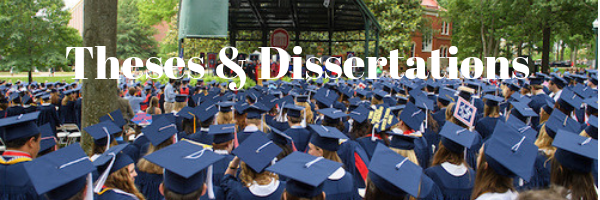
Date of Award
1-1-2024
Document Type
Dissertation
Degree Name
Ph.D. in Engineering Science
First Advisor
Samrat Choudhury
Second Advisor
Samrat Choudhury
Third Advisor
Shan Jiang
School
University of Mississippi
Relational Format
dissertation/thesis
Abstract
The processing–structure-chemistry–property (PSCP) linkage is a central to materials design. Identifying PSCP using conventional trial-and-error approach is a complex, time-consuming, and expensive task, due to the vast number of possible combinations. Machine learning (ML) can be applied to materials research to accelerate the study of PSCP. This dissertation summarizes the implementation of ML approaches to determine (a) chemistry-property linkage to design solid-solid heterointerfaces; (ii) processing-structure-property relationship for process optimization during additive manufacturing (AM).
In the study of solid/solid heterointerfaces using a density functional theory (DFT) and ML hybrid approach, the competition among features related to solute segregation energy ( ��������) at the Fe/Y2O3 interface were analyzed to accurately describe solute segregation behavior. Furthermore, ML models were developed to predict �������� at the Fe/Y2O3 interface within various system sizes, orientation relationships (OR), and dislocation conditions. ML also explored information transfer between these interfaces, effectively reducing the gap among them. In the design of novel fuel systems, DFT calculations were operated to investigate the immobilization of fission products (FPs) at U/(UN or UC) interfaces and U-Mo/(UN or UC) interfaces. Moreover, key features influencing segregation tendencies in these systems were identified by ML, contributing to the feature space for predicting ��������.
To explore additive manufacturing (AM), both internal (from experiments) and external (from literature) sources were utilized to assess key features affecting electrohydrodynamic (EHD) printing behavior through ML across various processing parameters and materials. The developed feature space was implemented into ML models to accurately predict the jetting mode and product size for new materials, thereby reducing the need for extensive experimentation. In the investigation of the fused deposition modeling (FDM) process using commercial Polylactic Acid (PLA) filament, processing conditions were designed to achieve the desired surface porosity. During the design, an ML algorithm based on uncertainty of prediction, adaptive design, was used to seek potential processing conditions corresponding to the target porosities. The predicted outcomes were experimentally validated, guiding the design of FDM processing conditions with high fidelity in a more efficient and cost-effective direction.
Recommended Citation
Lu, Yizhou, "Machine Learning Guided Materials Design and Manufacturing" (2024). Electronic Theses and Dissertations. 3019.
https://egrove.olemiss.edu/etd/3019