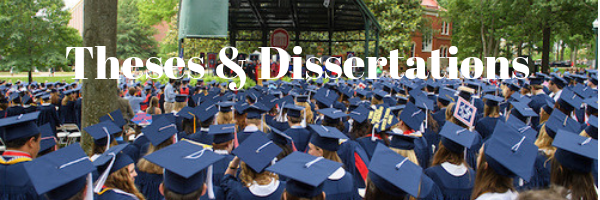
Date of Award
1-1-2024
Document Type
Dissertation
Degree Name
Ph.D. in Engineering Science
First Advisor
Hakan Yasarer
Second Advisor
Lance Yarbrough
Third Advisor
Yacoub Najjar
School
University of Mississippi
Relational Format
dissertation/thesis
Abstract
This dissertation introduces three innovative tools to advance groundwater analytics: the GRACE Downscaler, Synthetic Timeseries Generator, and a lightweight web-based service for deploying machine learning and physical models. These tools address critical challenges in groundwater management by enhancing the local applicability of GRACE satellite data, overcoming data scarcity through synthetic generation, and simplifying the deployment of complex hydrological models. The GRACE Downscaler provides a reproducible workflow for regional groundwater analysis, while the Synthetic Timeseries Generator creates opportunities for model development in data-poor regions. The web-based service demonstrates how advanced models like MODFLOW can be made accessible for rapid simulations and real-time scenario exploration. Unlike many existing frameworks, these tools prioritize accessibility and practical implementation alongside analytical power. This approach bridges the gap between advanced research models and real-world applications, setting a new paradigm for groundwater modeling and management. By providing user-friendly yet powerful tools, this work opens significant possibilities for future developments in the field, potentially transforming how water resource management decisions are made through more informed, data-driven processes.
Recommended Citation
Pulla, Sarva, "Open-Source Frameworks for Groundwater Analytics: Advancing Machine Learning Workflow Development and Deployment" (2024). Electronic Theses and Dissertations. 3026.
https://egrove.olemiss.edu/etd/3026