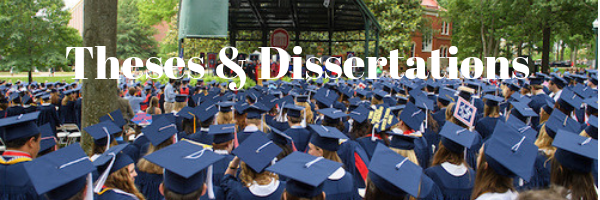
Date of Award
2016
Document Type
Dissertation
Degree Name
Ph.D. in Mathematics
Department
Mathematics
First Advisor
Hailin Sang
Second Advisor
Martial Longla
Third Advisor
Yixin Chen
Relational Format
dissertation/thesis
Abstract
We study the ideal variable bandwidth kernel density estimator introduced by McKay (1993) and the plug-in practical version of the variable bandwidth kernel density estimator with two sequences of bandwidths as in Ginè and Sang (2013).We estimate the variance of the variable bandwidth kernel density estimator. Based on the exact formula of the bias and the variance of the variable bandwidth kernel density estimator, we develop the optimal bandwidth selection of the true variable bandwidth kernel density estimator. Furthermore, we present the central limit theorem of the true variable bandwidth kernel density estimator. We also propose a new variable bandwidth kernel regression estimator and estimate the bias and propose the central limit theorems for its ideal and true versions. For the one dimensional case, the order of the bias and variance is same for the variable bandwidth kernel density estimator and for the proposed variable bandwidth kernel regression estimator. Since we use the order of the bias and variance to find the optimal bandwidth, the optimal bandwidth for these estimators are also the same. Comparing the integrated mean square error of the variable bandwidth kernel density estimator (the variable bandwidth kernel regression estimator) with the classical kernel density estimator (the Nadaraya-Watson estimator), we find that the variable bandwidth kernel estimators have a faster rate of convergence. Furthermore, we prove that these variable bandwidth kernel estimators converge to normal distribution.
Recommended Citation
Nakarmi, Janet, "On Variable Bandwidth Kernel Density And Regression Estimation" (2016). Electronic Theses and Dissertations. 678.
https://egrove.olemiss.edu/etd/678