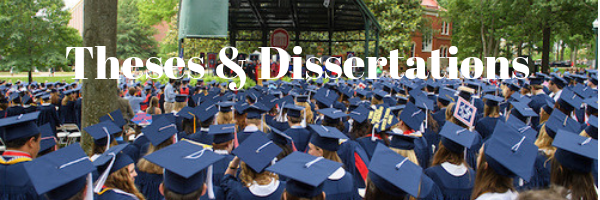
Date of Award
2016
Document Type
Thesis
Degree Name
M.S. in Engineering Science
Department
Electrical Engineering
First Advisor
Cristiane Q. Surbeck
Second Advisor
Andrew O'Reilly
Third Advisor
Yacoub Najjar
Relational Format
dissertation/thesis
Abstract
Many scientists and government agencies rely on Fecal Indicator Bacteria (FIB) to determine the risk of exposure to pathogens in water. If there is a presence of these indicator bacteria, pathogenic microorganisms may also be present. These bacteria in recreational water bodies pose a health threat to people if ingested during activities such as swimming or from the consumption of marine life. The most commonly tested FIBs are total coliforms, Fecal coliforms, Escherichia coli, Fecal streptococci, and enterococci. The measurement of bacteria can be time consuming (up to 48 hours) and expensive, creating a difficulty in warning the public of a potential risk. Ideally, a predictive model could determine the fib concentration in real-time, eliminating the current delays. Many analytical, statistical, process-based, and empirical models exist for water quality prediction, but produce a low level of precision and accuracy. Artificial Neural Network (ANN) models create a better model for predicting water resource variables because they are often capable of modeling complex systems of behavioral rules or underlying physical processes that are often difficult to simulate. An ANN is a computational model based on the biological neural networks. ANNs consists of processing units known as neurons or nodes that are joined by weighted connections. The connections are adjusted by determining an error quantity between the newly predicted output and the actual output and then applying the correction to each weighted node allowing the network to "learn” as it applies the correction for all data in the model. By modeling the ANN using easily recorded inputs to predict indicator bacteria, the concentration of bacteria can be provided almost instantaneously through the model. Due to the non-linear behaviors of most water quality parameters and the learning capabilities of ANNs, the development of a model could provide an adequate method for determining the risk for pathogen exposure in a timely manner. The study determined that ANN models performed well at predicting e. Coli concentrations that were around or greater than 126 cfu/100 ml. The results determined that focusing on specific locations for ANN models provided a higher accuracy.
Recommended Citation
Douglas, Christopher, "Bacteria Prediction For Recreational Waters Through The Use Of Artificial Neural Networks" (2016). Electronic Theses and Dissertations. 983.
https://egrove.olemiss.edu/etd/983