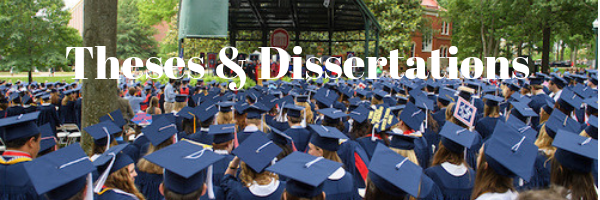
Date of Award
1-1-2024
Document Type
Thesis
Degree Name
M.S. in Engineering Science
First Advisor
Charles Walter
Second Advisor
Yixin Chen
Third Advisor
Thai Le
Relational Format
dissertation/thesis
Abstract
With the increasing use of AI in various applications, its ability to impact different communities is a crucial aspect to the advancement of humanity and technology. However, with its often unexplained properties, AI models could wreck havoc if loopholes are found. Adversarial attack algorithms have been shown to cause deep neural networks to fail on image classification and object detection tasks while being visually imperceptible to humans. Adversarial attacks cause out of distribution predictions and can cause the model to fail in unexpected ways.
Recommended Citation
Datta, Soumil, "On Inference-Time Adversaries in Object Detection Models" (2024). Electronic Theses and Dissertations. 2802.
https://egrove.olemiss.edu/etd/2802