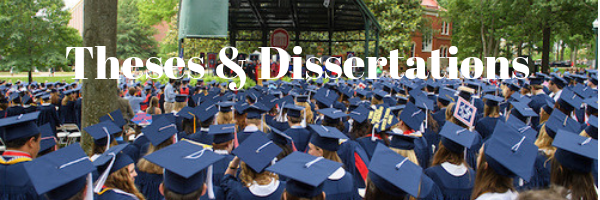
Date of Award
1-1-2024
Document Type
Dissertation
Degree Name
Ph.D. in Engineering Science
First Advisor
Thai Le
Second Advisor
Charles Walter
Third Advisor
Hailin Sang
School
University of Mississippi
Relational Format
dissertation/thesis
Abstract
Powerful but complex models have seen extensive adoption within recent years due to their performance across a wide range of tasks. However, this effectiveness has come at the cost of a limited understanding of how exactly these models make their decisions. This lack of understanding has justifiably slowed integration of these models within domains where serious consequences can result due to error like medicine or finance. In response, the discipline of explainable AI (XAI) has arisen to provide justification for why these models work the way they do. One of the most prominent tools in XAI has been the local surrogate model, a focused and simplified version of the complex model to be explained designed in a way that its architecture inherently restricts its complexity and so renders its decisions understandable. Simultaneously with the rise of surrogate methods comes the question of their effectiveness at accurately representing the workings of the complex model. One of the important attributes necessary to trust a surrogate model is robustness or stability, a property where insignificant changes to the surrogate model’s input result in correspondingly small changes to its output. Here we examine the robustness of one of the most popular XAI methods, LIME, then extend our analysis to the refinement of robustness estimates for any arbitrary XAI model that uses ranked lists of text-based data. We conclude by investigating fundamental attributes of how knowledge is stored within GPT-like models to provide a foundation for advancement beyond the limitations imposed by local surrogates.
Recommended Citation
Burger, Christopher, "Explainable AI for Neural Text Classification Models: Robustness and Knowledge Locality" (2024). Electronic Theses and Dissertations. 2925.
https://egrove.olemiss.edu/etd/2925