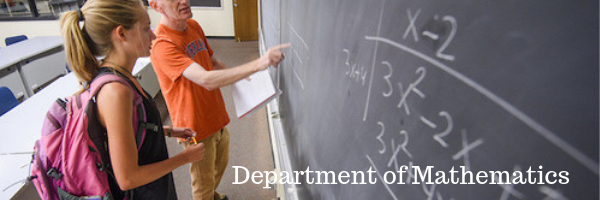
Faculty and Student Publications
Document Type
Article
Publication Date
1-1-2020
Abstract
© 2020 International Society for Bayesian Analysis Markov chain Monte Carlo (MCMC) methods are ubiquitous tools for simulation-based inference in many fields but designing and identifying good MCMC samplers is still an open question. This paper introduces a novel MCMC algorithm, namely, Nested Adaptation MCMC. For sampling variables or blocks of variables, we use two levels of adaptation where the inner adaptation optimizes the MCMC performance within each sampler, while the outer adaptation explores the space of valid kernels to find the optimal samplers. We provide a theoretical foundation for our approach. To show the generality and usefulness of the approach, we describe a framework using only standard MCMC samplers as candidate samplers and some adaptation schemes for both inner and outer iterations. In several benchmark problems, we show that our proposed approach substantially outperforms other approaches, including an automatic blocking algorithm, in terms of MCMC efficiency and computational time.
Relational Format
journal article
Recommended Citation
Nguyen, D., de Valpine, P., Atchade, Y., Turek, D., Michaud, N., & Paciorek, C. (2020). Nested Adaptation of MCMC Algorithms. Bayesian Analysis, 15(4), 1323–1343. https://doi.org/10.1214/19-BA1190
DOI
10.1214/19-BA1190
Accessibility Status
Searchable text